Transforming Drug Discovery Through AI
At Sinkove, we are dedicated to harnessing the power of AI to accelerate drug development process with synthetic images. Recently, our team collaborated with Pfizer on a groundbreaking research study demonstrating how AI can transform drug discovery through advanced image-based cell profiling. We use generative AI to create synthetic data to reduce biases derived from Inconsistent acquisition protocols in cell paiting.
Read Our Research
Check out our pre-print article written with our Pfizer collaborators detailing this groundbreaking research.
View the Pre-print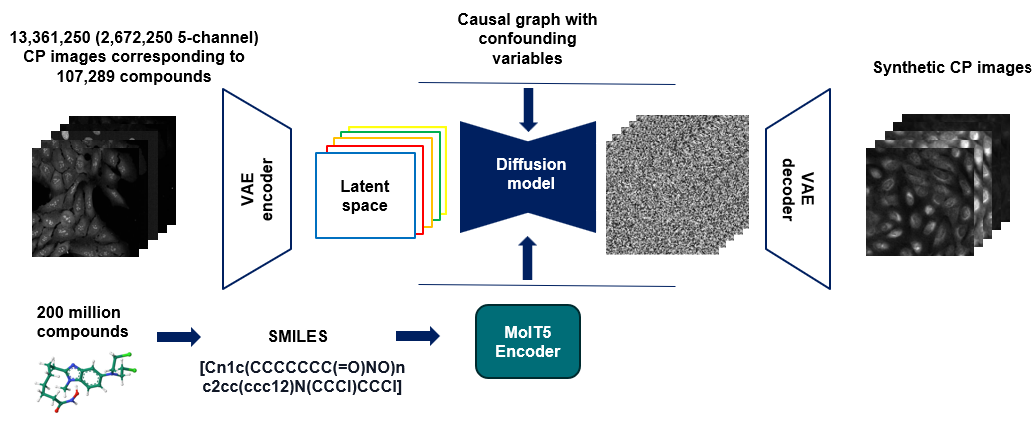
Understanding the Challenge - Biases
Cell Painting (CP) is a powerful microscopy technique used to capture detailed images of cells, revealing how they respond to different treatments or compounds. These images are vital for discovering how potential new drugs affect cell morphology and identifying mechanisms of action (MoA). However, experimental variability—such as differences in laboratory conditions, batches, or imaging techniques—can introduce significant bias, making it challenging to accurately interpret results and apply them to new compounds.
How AI Addresses These Challenges
Our solution is an advanced AI model called a confounder-aware latent diffusion model (LDM). Unlike traditional models, our AI learns to generate synthetic images of cells by explicitly accounting for confounding variables such as lab conditions and experimental setups. By incorporating a structural causal model (SCM) into our AI framework, we control for these biases, ensuring that the generated images reflect genuine biological effects rather than experimental noise.
Trained on an impressive dataset of over 13 million cell images and more than 107,000 compounds, our AI model also leverages chemical structure information encoded via SMILES representations. This powerful integration ensures our AI-generated images accurately reflect the biological impact of diverse chemical compounds.
Real Results from Synthetic Data
The benefits of using our AI-generated images are remarkable. Our confounder-aware AI model achieved state-of-the-art performance in predicting the mechanisms of action (MoA) and compound targets, significantly outperforming traditional and even batch-corrected real data.
Crucially, our AI excels not only in known scenarios but also shows impressive capabilities in accurately predicting biological effects for compounds never previously encountered during training. This opens exciting possibilities for exploring new chemical spaces and rapidly advancing drug discovery efforts.
Applications and Future Directions
At Sinkove, our mission continues to be the advancement of healthcare innovation through responsible and powerful AI tools. By augmenting real datasets with high-quality, AI-generated images, we provide researchers and clinicians with the tools they need to accelerate discovery, reduce bias, and deliver impactful health solutions more quickly and accurately. Together, we’re shaping the future of drug discovery—one AI-generated image at a time.