Did you know that synthetic images generated by AI can help medical classifiers remain accurate across different hospitals, patient demographics, and imaging techniques? A recent paper in Nature Medicine—"Generative Models Improve Fairness of Medical Classifiers Under Distribution Shifts" (Ktena et al., 2024)—offers compelling evidence that these methods can mitigate biases and improve diagnostic performance when real-world conditions diverge from the training data.
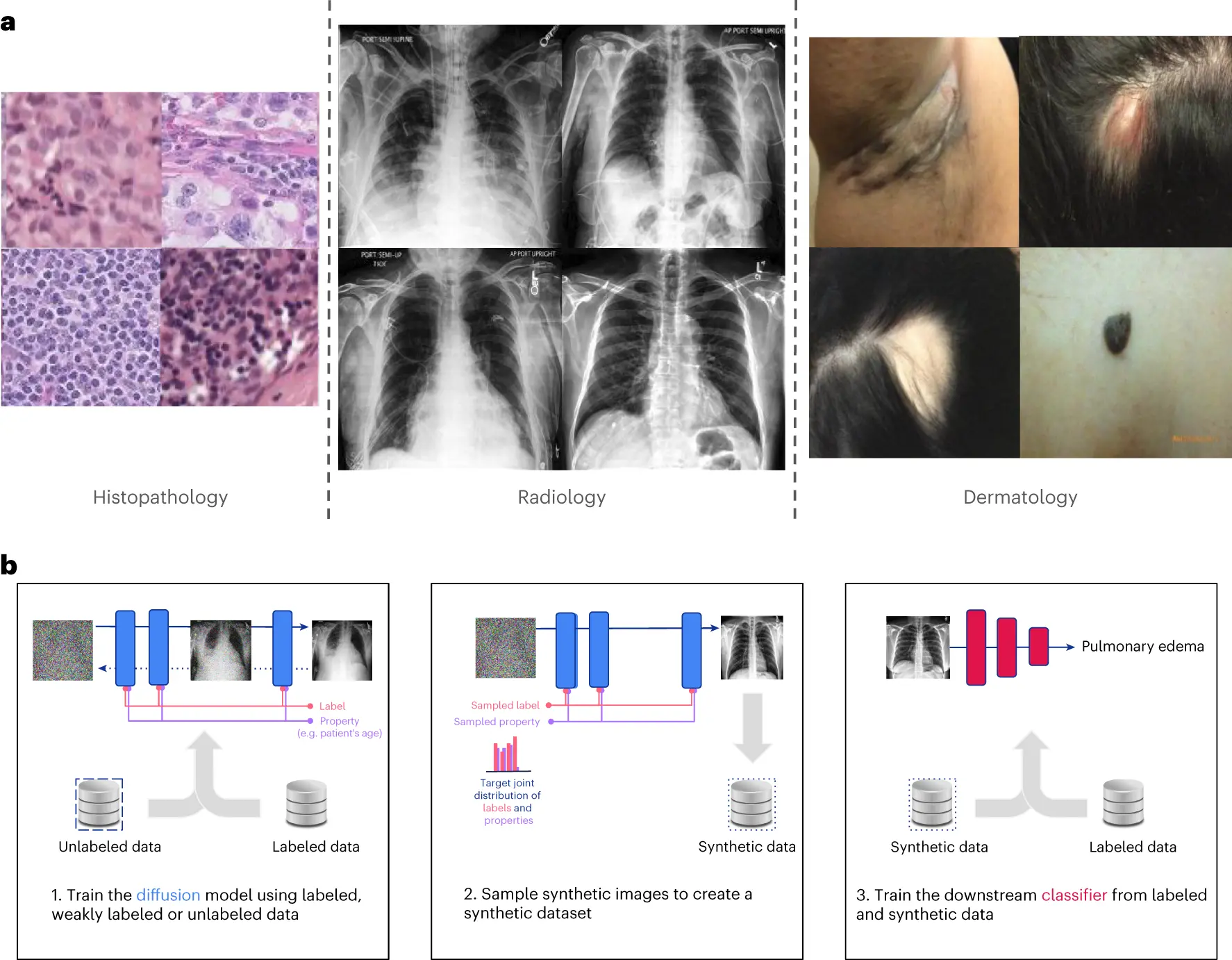
Context & Relevance
Distribution shifts—differences between training and real-world clinical data—often wreak havoc on the accuracy and fairness of machine learning models in medicine. Underrepresented patient subgroups (e.g., certain age ranges, ethnicities, or hospitals) further exacerbate these mismatches. This paper explores how generative AI (specifically diffusion models) can create realistic synthetic images that expand training datasets, reduce bias, and maintain robust performance across multiple medical imaging domains.
From Sinkove's perspective, this research is vital: our mission in the radiology and in silico trials space involves ensuring our AI solutions remain unbiased and clinically reliable, even under real-world data shifts.
Paper Analysis
Summary
Medical machine learning models often perform worse in real-world conditions compared to their training environments, especially when encountering demographic groups or imaging conditions not well represented in the original datasets. This paper from Nature Medicine demonstrates how generative AI models, particularly diffusion models, can generate synthetic medical images to effectively address data scarcity and demographic underrepresentation. The approach significantly improves the robustness, accuracy, and fairness of medical classifiers across diverse clinical scenarios, including histopathology, radiology, and dermatology.
Key Insights
Synthetic Data as Augmentation: Generative diffusion models successfully create realistic synthetic images, enriching training datasets with diverse examples, especially targeting underrepresented subgroups.
Fairness Across Groups: Synthetic data helps reduce performance gaps among different demographic groups, enhancing fairness.
Robustness to Distribution Shifts: Models trained with synthetic data demonstrate improved accuracy when tested under out-of-distribution conditions, such as different hospitals, demographics, or imaging techniques.
Label Efficiency: Generative models require fewer labeled examples, addressing scenarios where obtaining expert annotations is costly or impractical.
Experimental Setup
The study conducted extensive experiments across three medical imaging modalities:
• Histopathology: Diagnosed cancer metastases from lymph node images, using data from multiple hospitals with varying staining procedures.
• Radiology (Chest X-rays): Classified conditions such as cardiomegaly and pulmonary edema using images from CheXpert and ChestX-ray14 datasets, representing demographic and clinical variations.
• Dermatology: Evaluated skin conditions, particularly high-risk categories (e.g., melanoma), using images from datasets in the U.S., Australia, and Colombia, reflecting diverse patient populations.
Synthetic images generated by diffusion models were mixed with real images at optimized ratios (50% synthetic for histopathology, 100% synthetic for radiology, and 75% synthetic for dermatology) to train downstream classifiers. Evaluations measured both diagnostic performance and fairness improvements.
Conclusions
Generative diffusion models significantly enhance the fairness and robustness of medical AI classifiers by providing high-quality synthetic training data. This method effectively addresses performance disparities, particularly in scenarios involving limited or imbalanced datasets. It proves especially valuable in realistic clinical settings characterized by demographic diversity and varying imaging protocols.
Impact and Connection with Sinkove
This paper directly validates Sinkove's strategic focus on synthetic data generation for radiology and clinical trials. Our AI-driven digital twins and synthetic datasets offer healthcare providers and medical technology companies a robust way to mitigate bias and ensure consistent AI performance across diverse patient groups and clinical conditions. Adopting generative AI aligns with Sinkove's mission to accelerate equitable, precise, and efficient clinical innovation, reinforcing our value proposition to customers and investors.
This study underscores a key point: generative approaches, especially when thoughtfully implemented, can measurably enhance both accuracy and equity in medical AI. As always, real-world testing and multi-site collaborations remain critical next steps. But the evidence is strong: synthetic data can be the remedy for many distribution shifts plaguing today's healthcare algorithms.